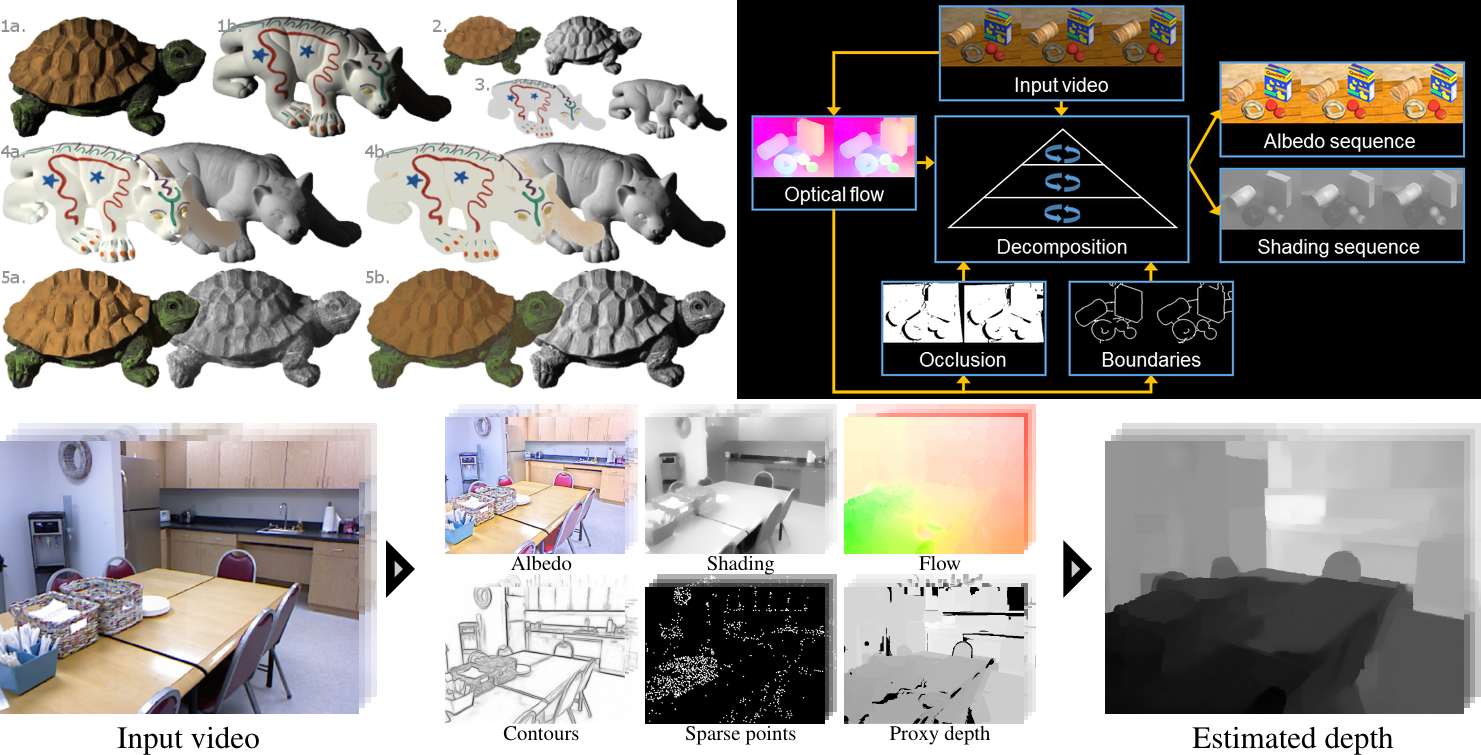
(Top left) Given a single image we decouple albedo and shading with a Global Sparsity prior on albedo. 1a-b: input images, 2,3: ground truth, 4a-b,5a-b: estimated albedo and shading with different settings a and b. (Top right) We extract temporally coherent albedo and shading sequences from video alone by exploiting physical properties derived from temporal variation in the video. (Bottom) We formulate the estimation of dense depth maps from video sequences as a problem of intrinsic image estimation.
Intrinsic images correspond to physical properties of the scene. It is a long-standing hypothesis that these fundamental scene properties provide a foundation for scene interpretation.
To decouple albedo and shading given a single image, we introduce a novel prior on albedo, that models albedo values as being drawn from a sparse set of basis colors [ ]. This results in a Random Field model with global, latent variables (basis colors) and pixel-accurate output albedo values. We show that without edge information high-quality results can be achieved, that are on par with methods exploiting this source of information. Finally, we can improve on state-of-the-art results by integrating edge information into our model.
While today intrinsic images are typically taken to mean albedo and shading, the original meaning includes additional images related to object shape, such as surface boundaries, occluding regions, and depth. By using sequences of images, rather than static images, we extract a richer set of intrinsic images that include: albedo, shading, optical flow, occlusion regions, and motion boundaries. Intrinsic Video [ ] estimates temporally coherent albedo and shading sequences from video by exploiting the fact that albedo is constant over time while shading changes slowly. The approach makes only weak assumptions about the scene and substantially outperforms existing single-frame intrinsic image methods on complex video sequences.
Intrinsic Depth [ ] steps towards a more integrated treatment of intrinsic images. Our approach synergistically integrates the estimation of multiple intrinsic images including albedo, shading, optical flow, surface contours, and depth. We build upon an example-based framework for depth estimation that uses label transfer from a database of RGB and depth pairs. We also integrate sparse structure from motion to improve the metric accuracy of the estimated depth. We find that combining the estimation of multiple intrinsic images improves depth estimation relative to the baseline method.