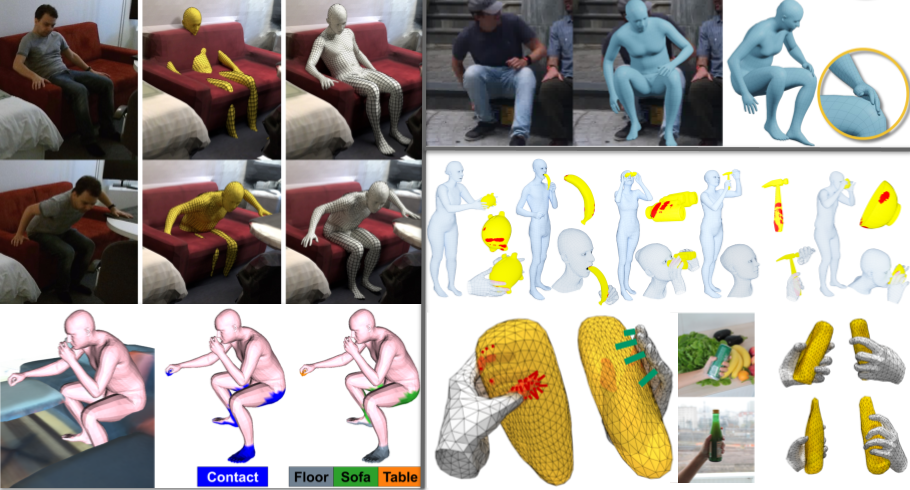
Contact plays an important role in understanding human-scene interactions and it takes many forms. Left: contact between the full body and furniture is used to improve monocular human pose and shape (HPS) estimation [ ] and to learn a body-centric representation for human-scene interaction [ ]. Top right: considering self-contact improves monocular HPS [ ]. Middle right: a dataset of whole-body grasps [ ]. Bottom right: contact between hands and hand-held objects helps reconstruct hands and objects jointly from images [ ].
Understanding and modeling human behavior requires capturing humans moving in, and interacting with, the world. Standard 3D human body pose and shape (HPS) methods estimate bodies in isolation from the objects and people around them. The results are often physically implausible and lack key information. We view contact as central to understanding behavior and therefore essential in human motion capture. Our goal is to capture people in the context of the world where contact is as important as pose.
To study this, we captured the PROX dataset [ ] using 3D scene scans and an RGB-D sensor to obtain pseudo ground truth poses with physically meaningful contact. Knowing the 3D scene enables more accurate HPS estimation from monocular RGB images by exploiting contact and interpenetration contraints.
Using the body-scene contact data from PROX, POSA [ ] learns a generative model of contact for the vertices of a posed body. We use this body-centric prior in monocular pose estimation to encourage the estimated body to have physically and semantically meaningful scene contacts.
TUCH [ ] explores HPS estimation with self-contact. We create novel datasets of images with known 3D contact poses or contact labels. Using these, and a contact-aware version of SMPLify-X [ ], we train a regression network using a modified version of SPIN [ ]. Not only is TUCH more accurate for images with self-contact, but also for non-contact poses.
To learn to regress 3D hands and objects from an image, we created the ObMan dataset by extending a robotic grasp simulator to MANO [ ] and rendering images of hands grasping many objects. We trained a network to regress both object and hand shape, while encouraging contact and avoiding interpenetration.
To address detailed whole-body contact during object manipulation we used mocap to create the GRAB dataset [ ]. GRAB goes beyond previous hand-centric datasets to capture actions like drinking where contact occurs between a cup and fingers as well as the lips.