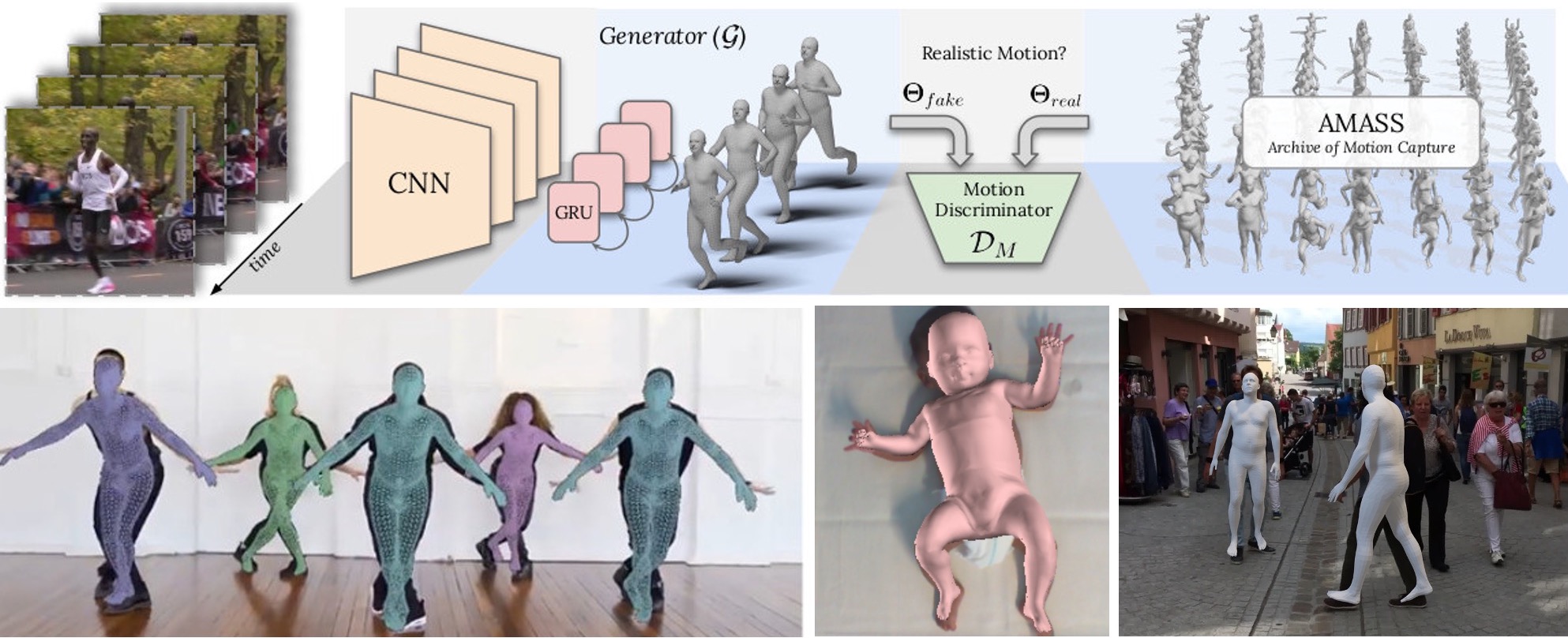
Top: VIBE regresses 3D human pose and shape from video using adversarial training by leveraging a large-scale human motion dataset (AMASS) to train a motion discriminator. Bottom left: output of VIBE. Bottom middle: SMIL estimates infant shape and motion from RGB-D videos to detect cerebral palsy. Bottom right: The 3DPW dataset combines IMU data with video to obtain high-quality pseudo ground truth 3D humans in video.
Humans are in constant motion. Interactions with the world and with each other involve movement. To capture, model, and synthesize human behavior we need to analyze it in video. Despite this, most methods for human 3D human pose and shape (HPS) estimation focus on single images. Intuitively, we should be able to exploit the regularity of human motion and the extra information provided by multiple video frames to improve HPS estimation compared to single-image methods. To that end, we are pursuing several lines or research to enable accurate markerless motion capture from unconstrained video "in the wild".
A key enabler of video-based analysis of motion is training data. To that end, we have exploited our 3D body models (SMPL, etc.) and MoSh, to create the large-scale AMASS dataset [ ] of human motions in a common 3D representation. We used an early version of this to generate the SURREAL dataset [ ], which contains rendered videos of people in motion. We used SURREAL, for example, to train methods to estimate the optical flow of people in video [ ]. We also used AMASS to train a network to estimate 3D human pose from a sparse set of IMUs [ ].
Synthetic datasets like SURREAL are not fully representative of real-world video. Consequently, we created the 3D Poses in the Wild dataset (3DPW) by combining IMU data with monocular video. IMUs are prone to drift but give 3D pose information. Videos give precise 2D alignment with image pixels but lack 3D. By combining these sources of information, 3DPW provides class-leading pseudo ground truth and is, consequently, widely used for training and evaluation.
To estimate 3D humans from video, we have pursued both optimization and regression approaches. Multi-View-SMPLify [ ] optimizes 3D pose over time using a generic DCT temporal prior. In contrast, VIBE [ ] uses a GRU-based temporal architecture to regress SMPL from video. VIBE exploits discriminative training using AMASS [ ] to help the network generate motions that resemble true human movement.
With SMIL [ ], we capture the motion of infants in RGB-D sequences but go further to use the sequences to learn the 3D shape model. By analyzing the movements of the infants, we provide an assessment related to cerebral palsy [ ].