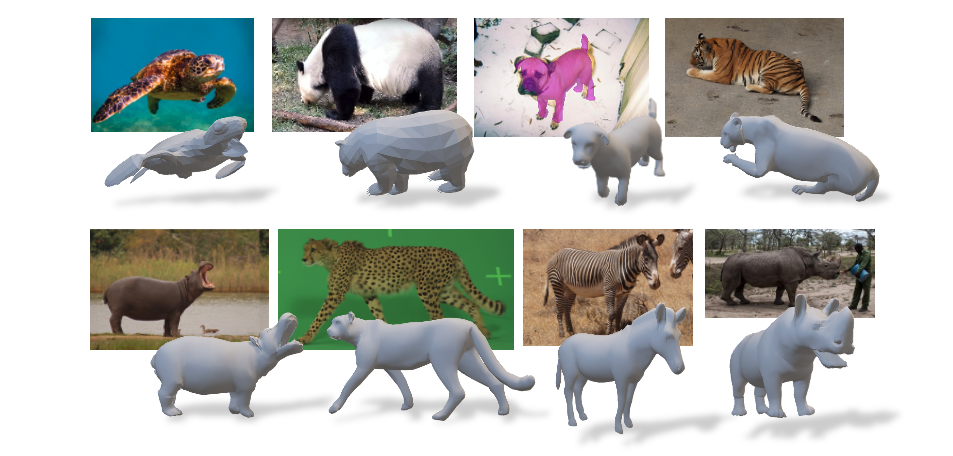
Our work estimates the shape and pose of animals from images and video. To achieve this, we learn 3D articulated shape models from 3D scans and 2D images.
In the past 20 years impressive advances have been made in capturing, modeling and tracking the human body in 3D, thanks to the availability of large amounts of 3D body scans and mocap data. Animals have received much less attention, despite many applications in biomechanics, biology, neuroscience, robotics, smart farming, and entertainment. The main reason for the lack of methods for the 3D modeling and tracking of animals is that the methods derived for the human body cannot be easily applied to animals: animals are not cooperative, cannot be brought to the lab in large numbers, and current scanners cannot be taken into the wild. It is also challenging to capture significant motion of animals using motion capture equipment.
In this project we develop methods to learn 3D articulated statistical shape models that can represent a wide variety of species in the animal kingdom, allowing intra- and inter-species analysis of 3D shape and the automatic and non-invasive assessment of animal shape and pose from images and video.
From scans of toy animals, we learn the SMAL (Skinned Multi Animal Linear) model [ ], a 3D articulated statistical shape model able to represent animal shapes for different species: big cats, dogs, cows, horses, zebras, and hippos. To capture animals outside the SMAL space, we developed SMALR (SMAL with Refinement) [ ]. SMALR estimates a detailed 3D textured mesh using a small set of uncalibrated, non-simultaneous images of the animal. We are also developing species-specific models for dogs, rats and horses exploiting different modalities.
Today animal motion is mostly captured indoors for domestic species with marker-based systems. We are exploiting our 3D articulated animal shape models to develop markerless motion capture systems that can capture the shape and articulated motion of wild animals in their natural environment. In this context, we have applied our technology to capture the shape, pose, and texture of the Grevy's zebra from in-the-wild images [ ] using a novel neural-network regressor. The approach learns the zebra shape space during training using a photometric loss.