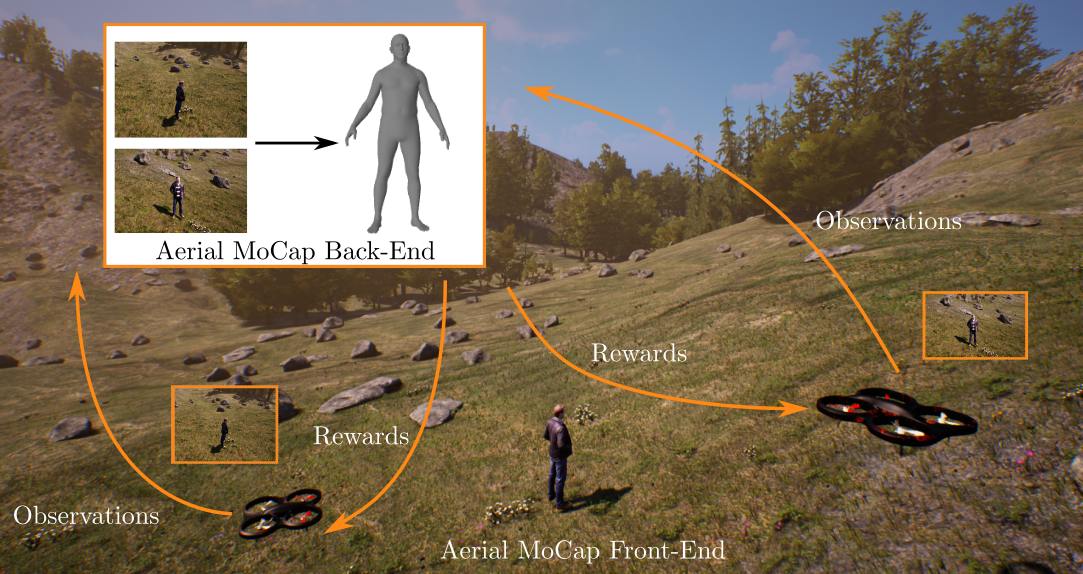
News: Our article related to this project is accepted to both IEEE RA-L and IROS 2020.
The source code and instructions to set up training and testing is now available here -- AirCap RL Github Repositiory
Article accepted to IEEE RA-L and IROS 2020, can be downloaded from here. Supplementary material associated to this paper can be found here. Therein the details of the network, reward curves, etc., are presented.
In this project, we introduce a deep reinforcement learning (RL) based multi-robot formation controller for the task of autonomous aerial human motion capture (MoCap). We focus on vision-based MoCap, where the objective is to estimate the trajectory of body pose and shape of a single moving person using multiple micro aerial vehicles. State-of-the-art solutions to this problem are based on classical control methods, which depend on hand-crafted system and observation models. Such models are difficult to derive and generalize across different systems. Moreover, the non-linearity and non-convexities of these models lead to sub-optimal controls. In our work, we formulate this problem as a sequential decision making task to achieve the vision-based motion capture objectives, and solve it using a deep neural network-based RL method. We leverage proximal policy optimization (PPO) to train a stochastic decentralized control policy for formation control. The neural network is trained in a parallelized setup in synthetic environments. We performed extensive simulation experiments to validate our approach. Finally, real-robot experiments demonstrate that our policies generalize to real world conditions.
The source code and instructions to set up training and testing is now available here