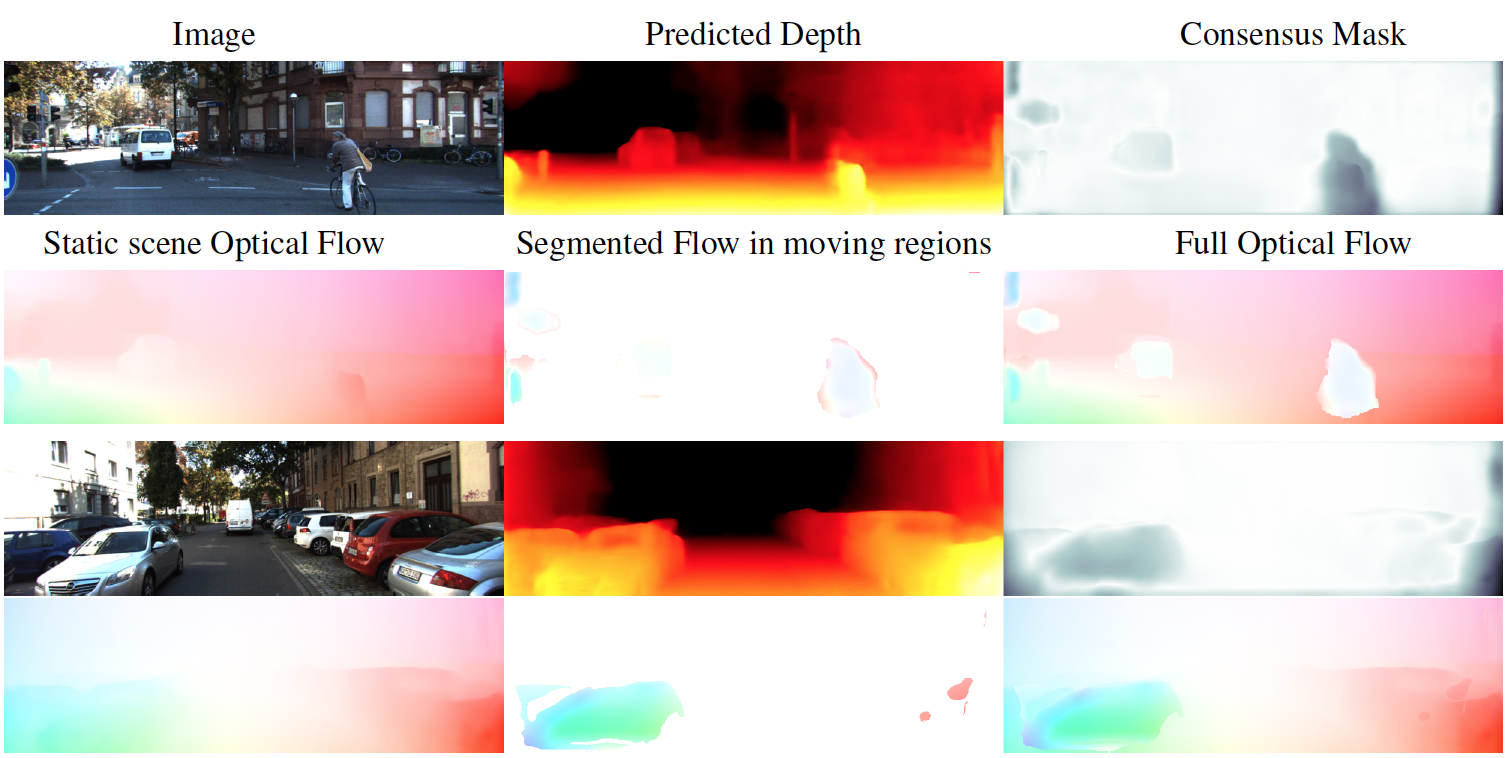
Unsupervised learning of depth, camera motion, motion segmentation (mask), and optical flow. Depth and camera motion gives rise to the flow in rigid regions. The networks learn to segment the non-rigid regions and compute flow in these. Everything is learned without supervision using a novel ``Competitive Collaboration'' method.
Humans and animals live in, and interact with, the 3D world around them. To understand humans then, we must understand the surfaces that support them and the objects with which they interact. To that end, we develop methods to estimate the structure and motion of the world from a single image, video, or multiple images. We approach this by combining unsupervised learning with physical knowlege of the world.
For moving scenes, we compute the optical flow representing the projection of the 3D motion field into the image. In doing so, we exploit the geometric structure of the problem to simplify it. If the scene is rigid and only the camera moves, then the optical flow is completely described by the depth of the scene and the camera motion. Real scenes, however, contain rigid structure and independently moving objects. To deal with this, we segment the scene into regions corresponding to the different types of motion. To do so we exploit different constraints that are both geometric and semantic.
In the latter case, we know that certain objects like animals and cars can move independently while others, like buildings, cannot. Additionally objects like the road are typically planar and hence their motion is simply modeled. Thus, a semantic segmentation of the scene, provides information about what motions may be present where. We argue that segmentation and motion estimation go hand in hand and we have explored methods to do both in a coupled way.
Our most recent work focuses on the unsupervised learning of motion, scene depth, camera motion, and segmentation. Training exploits competitive collaboration in which different neural networks vie to explain the motion in the scene. To make this work, the physical constraints about motion in rigid scenes are critical. This geometric information allows us to learn depth from a single image without supervision. Motion makes this possible because scene structure is constant over time, allowing motion over time to inform the network about scene depth.
This work demonstrates that classical physical and geometric constraints that we already know about the world and its motion are not only compatible with deep learning but can play a critical role in enabling unsupervised learning. In a sense, the physics of the world provides a form of pre-existing supervision.
Our ongoing work combines people, scenes, and unsupervised learning to derive coherent explanations of the 3D world. We posit that the joint estimation of people and scenes will improve both.