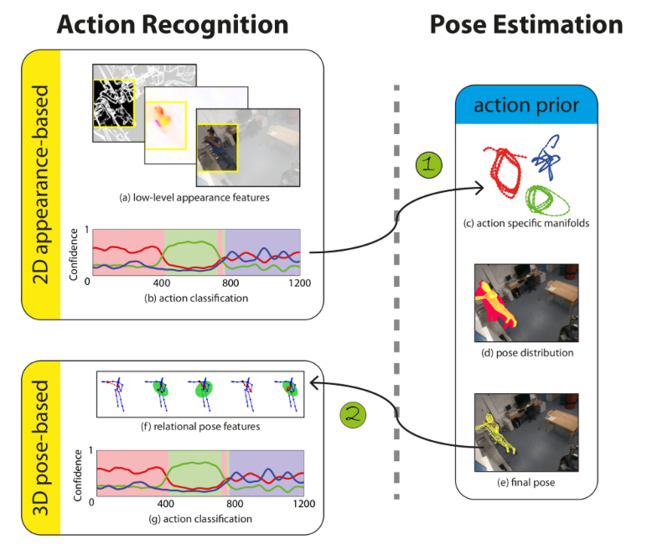
Action recognition can be performed based on low-level appearance features (a) such as color, optical flow, and spatio-temporal gradients or on features derived from the human pose (f). We have shown that action recognition benefits from human poses, but also that pose estimation can benefit from action recognition. For instance, outputs of the 2D action recognition (b) can be used as a prior distribution (c) for 3D pose estimation (d) (Arrow 1). Vice-versa, 3D pose-based action recognition (g) can be performed based on pose-based features (f) extracted from the estimated poses (e) (Arrow 2).
Vision-based human motion analysis attempts to understand the movements of the human body using computer vision and machine learning techniques. The movements of the body can be interpreted on a physical level through pose estimation, i.e., reconstruction of the 3D articulated motions, or on a higher, semantic level through action recognition, i.e., understanding the body's movements over time. While the objectives of the two tasks differ, they share a significant information overlap. For instance, poses from a given action tend to be a constrained subset of all possible configurations within the space of physiologically possible poses. Therefore, many state-of-the-art pose estimation systems use action-specific priors to simplify the pose estimation problem. At the same time, pose information can be a very strong indicator of actions.
Given that human pose estimation and action recognition are such closely intertwined tasks, information from one task can be leveraged to assist the other and vice versa. Therefore, we advocate in this project the use of information from action recognition to help with pose estimation and vice versa for the following reasons. First, using the results of an action classifier is a simple way to bring together many single-activity priors for pose estimation in multi-activity sequences. Secondly, pose-based action recognition has several advantages. For example, pose representations suffer little of the intra-class variances common in appearance-based systems; in particular, 3D skeleton poses are viewpoint and appearance invariant, such that actions vary less from actor to actor. Furthermore, using pose-based representations greatly simplifies learning for the action recognition itself, since the relevant high-level information has already been extracted.
To demonstrate the advantage of coupling the closely intertwined tasks of action recognition and pose estimation, we have developed a framework that jointly optimizes over several low-dimensional spaces that represent poses of various activities. Beyond that, unobserved pose variations or unobserved transitions between actions are resolved by continuing the optimization in the high-dimensional space of all human poses. Our experiments have shown that this combination is superior compared to optimization in either space individually.
For action recognition, 3D pose-based features have been shown to be more successful at classifying the actions than 2D appearance-based features. The same has been shown to be true even when the pose-based features were extracted from the estimated poses of the developed pose estimation system, indicating that the quality of estimated poses with an average error between 42mm-70mm is sufficient enough for reliable action recognition.
To advance vision-based human motion analysis beyond isolated actions and poses, our current research is focused on integrating contextual information, either from the environment or objects. Environmental context, e.g., the type of scene or even specific locations within a scene can provide strong indicators to the types of actions and therefore poses which can be expected. Furthermore, interactions with objects can often be the defining characteristic of an action and having a better understanding of human-object interactions would lead to improved recognition on high-level actions.