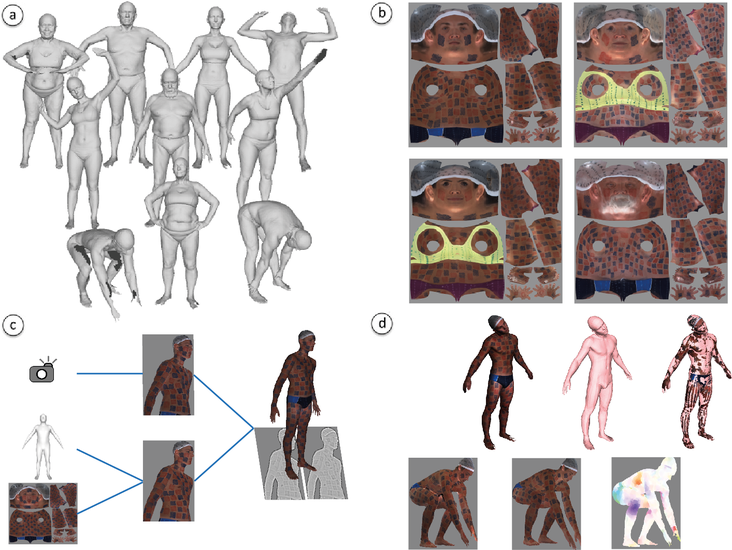
FAUST dataset. (a) FAUST contains 300 real human scans of 10 different subjects in 30 different poses, acquired with a high-accuracy 3D multi-stereo system. (b) To achieve full-body ground-truth correspondence between scans, we paint the subjects with a high- frequency texture pattern. (c) Every scan is brought into alignment with a 3D human body model. Our technique exploits texture information, bringing the model into registration with multi-camera image data using a robust matching term. (d) We evaluate the quality of our alignments in terms of both geometry and color consistency, discarding correspondences that are not reliable enough to be considered "ground truth".
New scanning technologies are increasing the importance of 3D mesh data and the need for algorithms that can reliably align it. Surface registration is important for building full 3D models from partial scans, creating statistical shape models, shape retrieval, and tracking.
The problem is particularly challenging for non-rigid and articulated objects like human bodies. While the challenges of real-world data registration are not present in existing synthetic datasets, establishing ground-truth correspondences for real 3D scans is difficult.
We propose a new dataset called FAUST (Fine Alignment Using Scan Texture), containing 300 real, high-resolution human scans of 10 different subjects in 30 different poses, with automatically computed ground-truth correspondences.
Scans are acquired through a 3D multi-stereo system; each scan is a high-resolution, triangulated, non-watertight mesh. Compared to synthetic meshes, FAUST scans present much more challenging features: missing data, different topologies, high resolution, realistic deformations, self contacts.
We define ground-truth correspondences between these scans by bringing each scan into alignment with a common template mesh, using a novel technique that exploits both 3D shape and surface texture information.
To achieve full-body ground-truth correspondence between meshes, we paint the subjects with a high-frequency texture pattern and place textured markers on key anatomical locations.
Our alignment technique estimates scene lighting and surface albedo, and uses the albedo to construct a high-resolution textured 3D model. The model is brought into registration with multi-camera image data using a robust matching term. Texture information adjusts vertex placement mostly in smooth 3D areas (like the stomach and back), complementing the partial or ambiguous information provided by the shape and improving intra-subject correspondences between scans.
We verify the quality of our alignments both in terms of geometry and color, and ensure that the ground-truth correspondences we define are accurate within 2mm.
While we exploit painting to provide reliable dense intra-subject correspondences, between different subjects we define only a set of sparse ground-truth correspondences. Neither the natural texture of different people, nor our painted texture, can be matched across subjects. And in general, a correspondence across different body shapes may not be well defined. We take an approach that is common in the anthropometry and motion capture communities of identifying key landmarks on the body, and we use these for sparse correspondence -- drawing a set of 17 easily identifiable landmarks on specific body points where bones are palpable.
FAUST is subpided into a training and a test set. The training set includes 100 scans (10 per subject) with their corresponding ground-truth alignments. The test set includes 200 scans. The FAUST benchmark defines 100 preselected scan pairs, partitioned into two classes – 60 requiring intra-subject matching, 40 requiring inter-subject matching.
The dataset and evaluation website will be available soon at http://faust.is.tue.mpg.de.