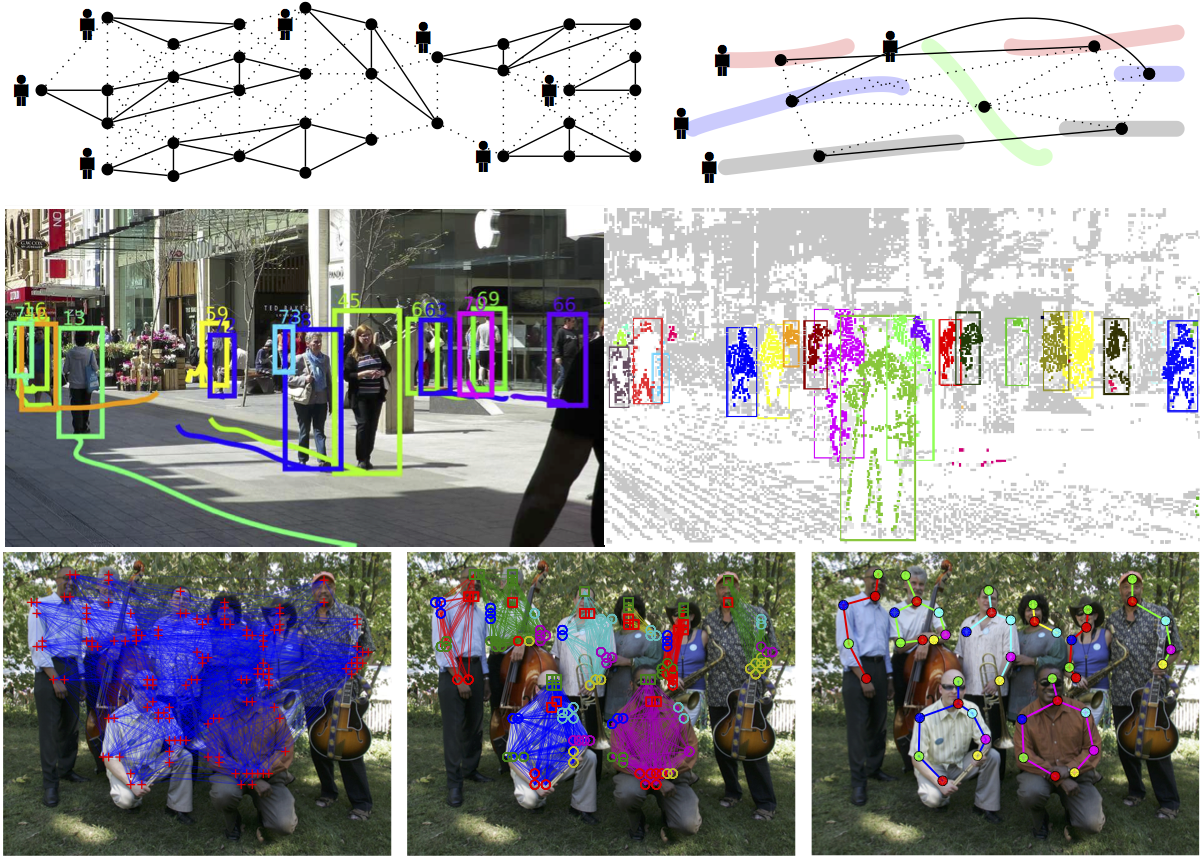
Top row illustrates the hierarchical correlation clustering formulation for multi-person tracking [ ]. A dotted line indicates that the edge is a cut. The detection graph is partitioned into 7 components, indicating 7 people (top left), which are associated by the global clustering, resulting in 4 persons (top right). Middle row shows qualitative results of tracking and segmentation on the MOT16 benchmark. The solid line under each bounding box indicates the lifetime of the track. Bottom row illustrates the Deepcut model [ ] for multi-person pose estimation. Initial detections (bottom left) and pairwise terms between all detections are jointly clustered and each part is labeled corresponding to its part class. Bottom right shows the predicted pose sticks.
People are often a central element of visual scenes. It has been a long-standing goal in computer vision to develop computational models that enable machines to detect crowds of people, analyze their motion and poses, infer their actions and reason about the consequences. Our research addresses a wide range of challenges in visual understanding of people in real-world crowded scenes. These include multi-person tracking [ ] [ ], multi-person pose estimation [ ], segmentation [ ] and person re-identification [ ].
For multi-target tracking, our work [ ] proposed to link, cluster and track targets jointly across space and time. We defined a novel mathematical abstraction for tracking in the form of a minimum cost multicut problem. In order to avoid that distinct but similar looking targets are assigned to the same track, we formulated tracking as a minimum cost lifted multicut problem [ ].
Our work [ ] presented a novel method to re-identify people in different images, where a second-pooling method is utilized to fuse the feature maps from the pose and the appearance estimator. The method significantly advanced the state-of-the-art on many challenging public benchmarks.
This work forms a foundation for our ongoing work on estimating detailed 3D motions of people in crowded scenes.