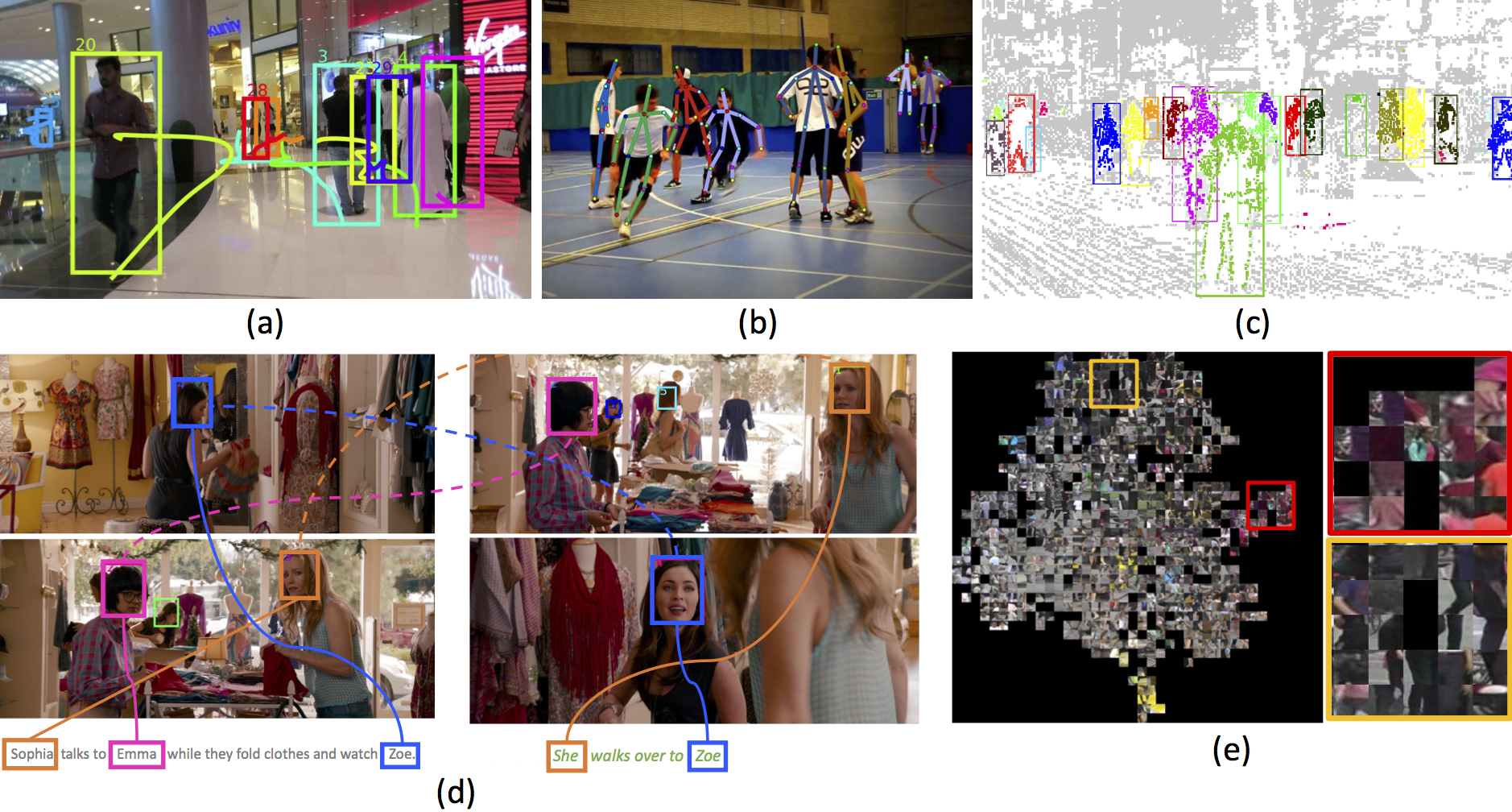
Our research covers a wide range of applications that involve visual understanding of people in real-world crowded scenes, including (a) people tracking [ ][ ], (b) multi-person pose estimation [ ], (c) segmentation [ ], (d) visual grounding [ ] and (e) person re-identification [ ].
Our goal is to understand the process of perception, to learn the representations that allow complex reasoning about visual input, inferring actions and predicting their consequences. We seek fundamental principles, algorithms and implementations for solving this task. In the past two years, we have made significant progress in this regard in terms of learning holistic scene representations, discovering rigorous mathematical abstractions, proposing efficient learning and inference techniques and advancing the state-of-the-art performance of various vision tasks in real-world diverse settings.
Efficient and scalable learning and optimization. A significant part of our research agenda focuses on using optimization methods to solve challenging real-world vision tasks. We were the first to formulate multi-target tracking as a Minimum Cost Multicut Problem and a Minimum Cost Lifted Multicut Problem [ ]. We further developed two efficient local search heuristic approximations [ ], which can propose solutions in a reasonable amount of time, while achieving state-of-the-art accuracy for complex vision tasks. A long-term goal is to automate the learning and inference process and make it more accessible for large-scale real-world settings.
Understanding people in unconstrained scenes. We explore many aspects of visual understanding of people in real-world crowded scenes, including people detection, people tracking [ ][ ], multi-person pose estimation [ ], segmentation [ ], person re-identification [ ] and human activity analysis [ ].
Holistic scene perception. Our third thrust focuses on learning holistic scene representations. Our fundamental assumption is that robust machine perception involves learning holistic scene representations and predicting interdependent output variables. We proposed a novel method to learn holistic representations jointly from video and language [ ]. Our model learns to generate descriptions and jointly ground (localize) the mentioned characters while also performing visual co-reference resolution between pairs of consecutive sentences/clips in a movie. Our long-term goal is to study the algorithmic foundations enabling machines to learn holistic representations from different levels of visual granularity and multiple sensory inputs such as images and language.
Group Leader
PhD Student
Qianli Ma (with Michael Black)
Visitor
Yan Zhang Oct 2018 - March 2019
Master Student
David Hoffmann (with Dimitris Tzionas) April 2018 - present
Past Visitor
Jie Song June 2017 - December 2017