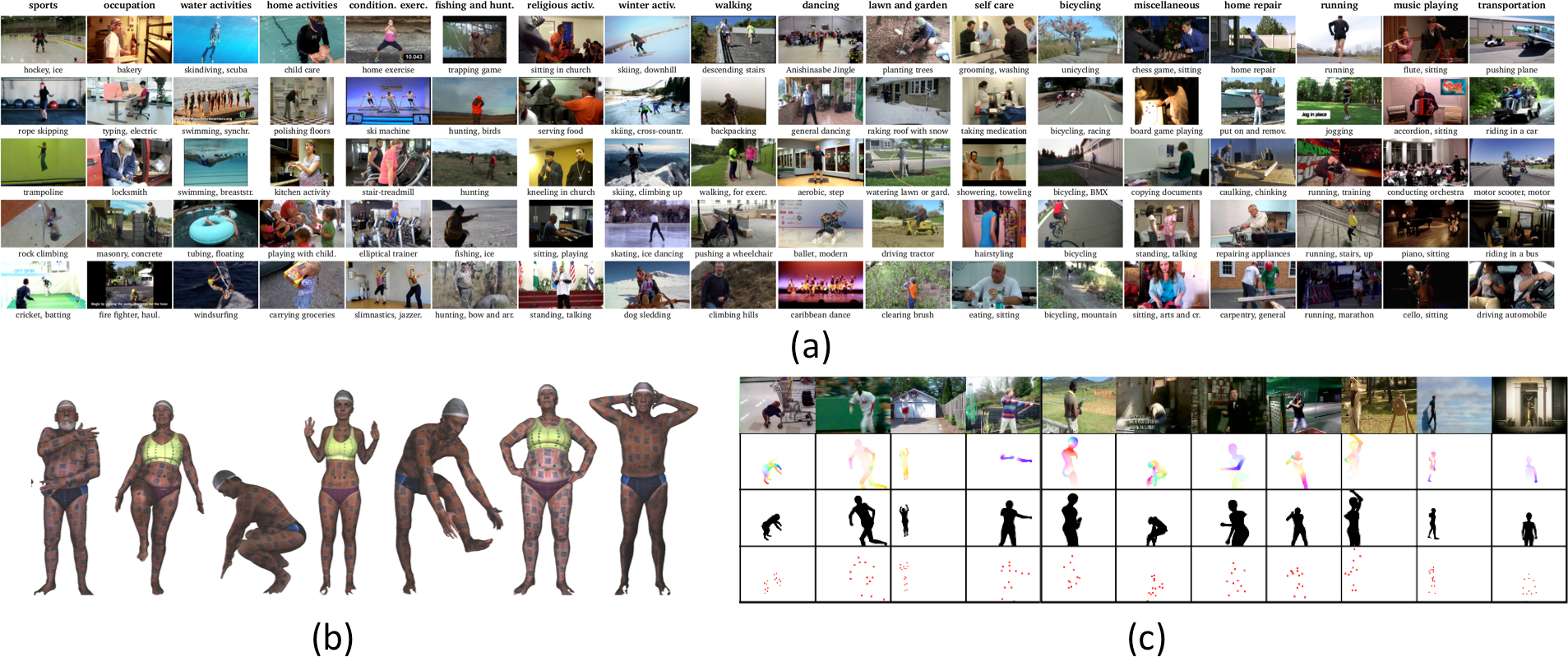
We propose novel challenging datasets for human pose estimation, 3D mesh registration and action recognition: a) MPII Human Pose, including around 25000 images of over 40000 people with annotated 2D body joints; b) FAUST, collecting 300 real human body scans with automatically computed ground-truth correspondences; c) J-HMDB, a dataset for action recognition with annotated human joints, segmentation, and optical flow.
Human pose estimation, 3D mesh registration and action recognition techniques have made significant progress during the last years. However, most existing datasets to evaluate them are inadequate for capturing the challenges of real-world scenarios. We introduce novel datasets and benchmarks, all publicly available for research purposes.
In [ ], we describe the datasets currently available for pose estimation and the performance of state-of-the-art methods on them. In [ ], we introduce a novel benchmark for pose estimation, "MPII Human Pose", that makes a significant advance with respect to previous work in terms of diversity and difficulty. It includes around 25000 images containing over 40000 people performing more than 400 different activities. We provide a rich set of labels including body joint positions, occlusion labels, and activity labels. Given these rich annotations we perform a detailed analysis of the leading human pose estimation approaches, gaining insights for the successes and failures of these methods.
FAUST [ ] is the first dataset for 3D mesh registration providing both real data (300 human body scans of different people in a wide range of poses) and automatically computed ground-truth correspondences between them. We define a benchmark on FAUST, and find that current shape registration methods have trouble with this real-world data.
With the "Joints for the HMDB" dataset (J-HMDB) we focus on action recognition [ ]. We annotate complex videos using a 2D "puppet" body model to obtain "ground truth" joint locations as well as optical flow and segmentation. We evaluate current methods using this dataset by systematically replacing the input to various algorithms with ground truth. This enables us to discover what is important -- e.g., should we improve flow algorithms, or enable pose estimation? We find that high-level pose features greatly outperform low/mid level features; in particular, pose over time is critical. Our analysis and the J-HMDB dataset should facilitate a deeper understanding of action recognition algorithms.