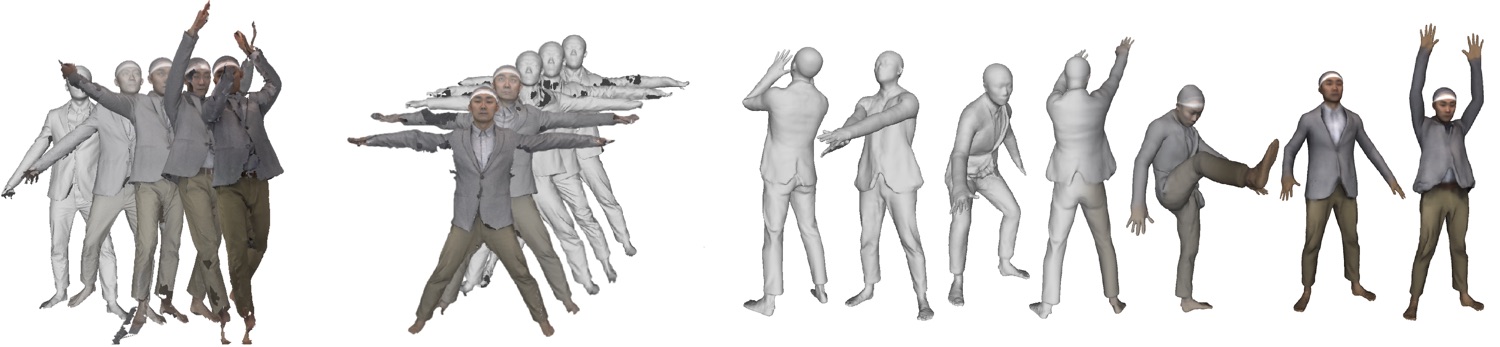
SCANimate learns an implicit 3D model of dressed humans from raw 3D scans.
Our approach to understanding humans and their behavior is grounded on 3D models of the body and its movement. Such models facilitate reasoning about human-object interaction, contact, social touch, and emotion. They make explicit what is implicit in images -- the form of the body and its relationship to the 3D world. Consequently, we are developing ever more accurate and detailed models of the human body.
Specifically, we learn realistic 3D models of human body shape and pose deformation from thousands of detailed 3D scans. We have built many models, but SMPL has become the de facto standard for research on human pose. To go beyond SMPL, we have learned a face model (FLAME) using a novel dataset of 4D facial sequences. FLAME captures realistic 3D head shape, jaw articulation, eye movement, blinking, and facial expressions. Similarly, we developed MANO, a 3D hand model learned from around 2000 hand scans of different people in many poses. We have combined SMPL, FLAME and MANO into a single model, SMPL-X, resulting in an expressive model of humans that can be animated and fit to data. Within the SMPL family of models, we have also learned models of soft-tissue deformation (DMPL), infants (SMIL) and animals (SMAL).
These classical models, based on triangulated meshes, however, are not flexible enough to easily model complex clothing and changes in topology. Consequently, we are developing 3D articulated models of clothed humans based on implicit functions. These are neural networks that characterize occupancy in 3D space or the signed distance to the surface of the person. These are highly flexible models and we are developing the tools to learn them from scans and images.
We are also pursuing 3D models based on point clouds. Like implicit shape models, these allow complex and varying topology but have the advantage of being fast to render and compatible with existing graphics tools.