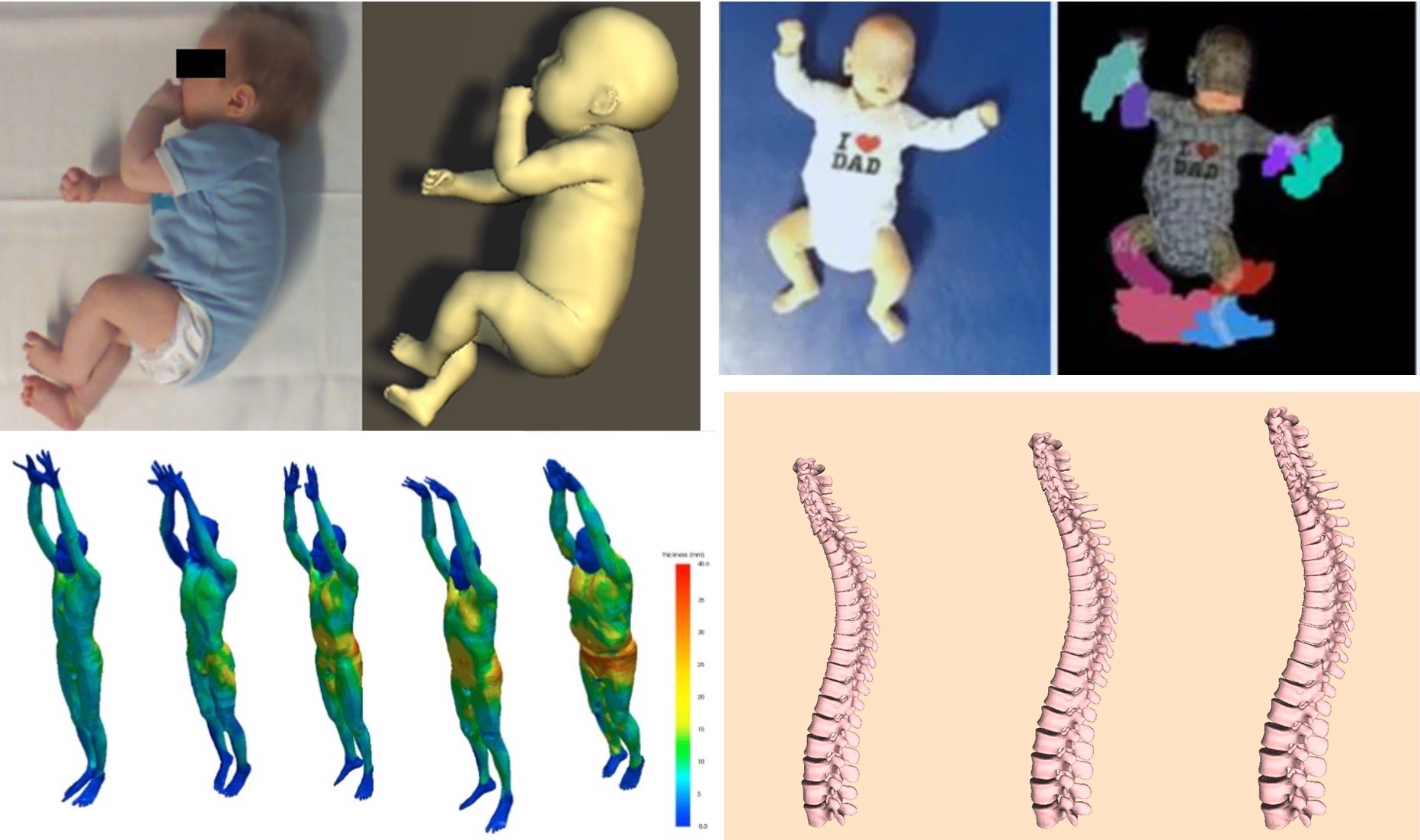
Top left: We learn an infant body model called SMIL from RGB-D sequences and use this to recover the shape and pose of freely moving infants. Top right: We use SMIL to track infant motion to assist with early diagnosis of cerebral palsy. Bottom right: Looking inside the body, we learn a model of spine shape and curvature. Bottle left: We align 3D bodies to MRI, segment the subcutaneous adipose tissue, and illustrate its thickness with a color code.
Body shape and movement are related to human health. Using our 3D models of body shape we analyze movement and shape to create non-invasive and deployable methods for analyzing human health.
For example, if Cerebral Palsy (CP) is detected early, there are effective therapies to minimize the impact in later life. CP can be diagnosed in infants based on their spontaneous, undirected movements. Unfortunately, this requires expert training that is not widely available. By automatically tracking infant movement, we can automate the early detection of CP. Methods for tracking adult 3D motion, however, do not work for infants due to their very different body shape.
Since we lack 3D scans of infants, we developed a novel method that learns an infant body shape model, called SMIL, directly from low quality, incomplete, RGB-D scan sequences and deployed this in hospitals where we scanned over 30 infants [ ]. Using SMIL we track spontaneous infant movements in RGB-D sequences and train a method to automatically recognize pathological movements [ ].
We also train methods to predict what is inside the body by observing the outside. Take, for example, the distribution of body fat. Visceral adipose tissue is correlated with disease while sub-cutaneous adipose tissue is relatively benign. We are developing methods to estimate this fat distribution purely from the surface shape of the body. To that end, we fit our 3D body models to full-body MRI scans [ ] to model both the external surface and the subcutaneous fat layer.
To make predictions of the body composition of a person from a surface scan, a main challenge lies in the variance of the body shape due to the pose. In collaboration with the University of Hawai'i Cancer Center (US) we show how SMPL allows us to factor out pose variations from surface scan data, improving the accuracy of the body composition estimation [ ].