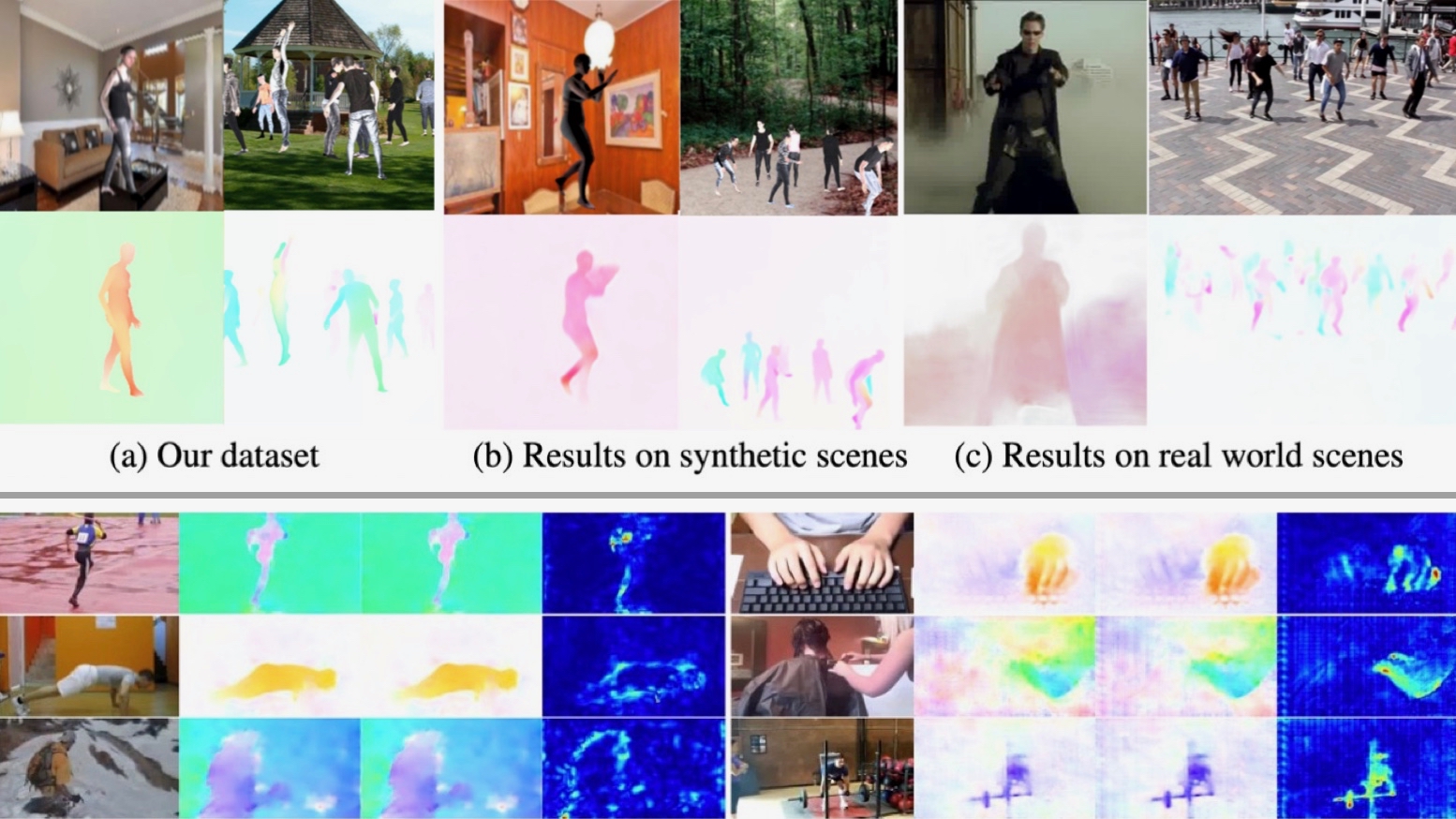
(Top) We learn human flow [ ] from synthetically generated flow fields and find that this generalizes to real videos of human movement. (Bottom) We fine tune an optical flow algorithm to produce flow that improves action recognition [ ]. (Left columns) SpyNet. (Right columns) FlowNet. In each set, left to right: first image in sequence, original flow, flow when trained on action recognition, differences in the flow are focused on the human action.
Understanding human action requires modeling and understanding human movement. While we mostly focus on 3D human movement, what is directly observable in videos is the 2D optical flow. Previous work has shown that flow is useful for action recognition and, consequently, we explore how to better estimate human flow and improve action recognition.
Specifically, we train a neural network to compute single-human [ ] and multi-human [ ] optical flow. To enable this we create a new synthetic training database of image sequences with ground-truth human optical flow. For this we use the 3D SMPL body model, motion-capture data, and computer graphics to synthesize realistic flow fields; this effectively extends the SURREAL dataset [ ]. We then train a convolutional neural network (SpyNet [ ]) to estimate human optical flow from pairs of images.
The new network is more accurate than a wide range of top methods on held-out test data and generalizes well to real image sequences. When combined with a person detector/tracker, the approach provides a full solution to the problem of 2D human flow estimation.
Most of the top-performing action-recognition methods use optical flow as a ``black box'' input. In [ ], we take a deeper look at the combination of flow and action recognition, and find that: 1) optical flow is useful for action recognition because it is invariant to appearance, 2) flow accuracy at boundaries and for small displacements is most correlated with action-recognition performance, 3) training optical flow needs to minimize classification error instead of the popular end-point-error (EPE) to improve action recognition, and 4) optical flow learned for action recognition differs from traditional optical flow mostly inside and at the boundary of human bodies.