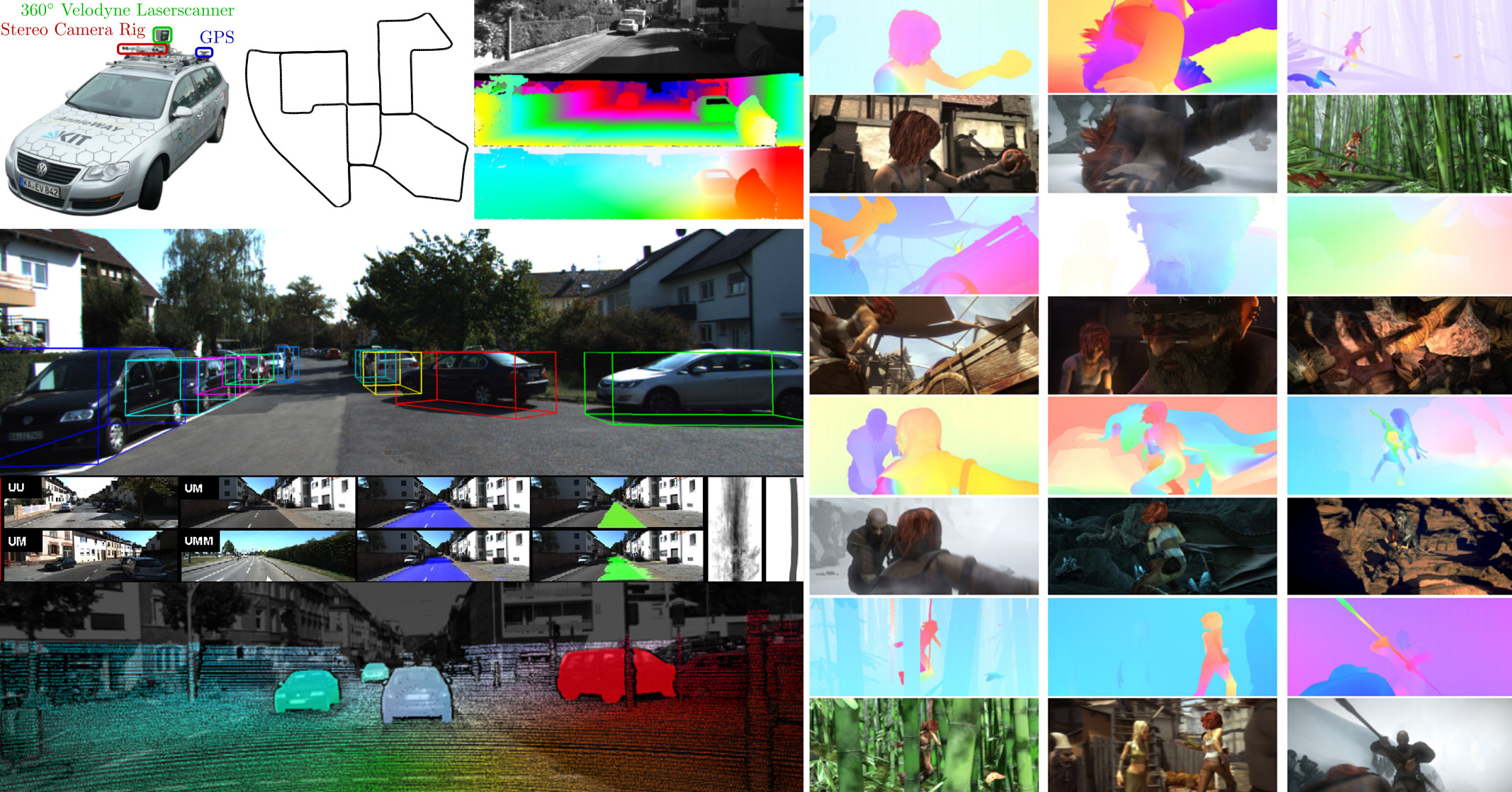
The KITTI Vision Benchmark Suite (left) and the MPI Sintel Benchmark (right) provide ground truth data and evaluation servers for benchmarking vision algorithms. So far, more than 400 methods have been evaluated on our benchmarks.
While ground truth datasets spur innovation, many current datasets for evaluating stereo, optical flow, scene flow and other tasks are restricted in terms of size, complexity, and diversity, making it difficult to train and test on realistic data. For example, we co-authored the Middlebury flow dataset [ ], which arguably set a standard for the field but was limited in terms of complexity.
In [ ], we took advantage of an autonomous driving platform to develop challenging real-world benchmarks for stereo, optical flow, scene flow, visual odometry/SLAM, 3D object detection, 3D tracking and road/lane detection. Accurate ground truth is provided by a Velodyne laser scanner and a GPS localization system. Our datasets are captured by driving around a mid-size city of Karlsruhe, in rural areas and on highways with up to 15 cars and 30 pedestrians visible per image. For each of our benchmarks, we also provide a set of evaluation metrics and a server for evaluating results on the test set. Our experiments showed that moving outside the laboratory to the real world was critical. We continue to develop new ground truth to push the field further.
In [ ], we proposed a novel optical flow, stereo and scene flow data set derived from the open source 3D animated short film Sintel. We extracted 35 sequences displaying different environments, characters/objects, and actions and showed that the image and motion statistics of Sintel are similar to natural movies. Using the 3D source data, we created an optical flow data set exhibits important features not present in previous datasets: long sequences, large motions, non-rigidly moving objects, specular reflections, motion blur, defocus blur, and atmospheric effects. We released the ground truth optical flow for 23 training sequences and withheld the remaining 12 sequences for evaluation purposes. When released in 2012, the best methods had an average endpoint error of around 10 pixels. The dataset has focused the community on core problems and only 3.5 years later, there are over 70 methods evaluated on the benchmark with the best methods are approaching 5 pixels in error.