Semantic Scene Understanding
- Perceiving Systems
- Semantic Scene Understanding
- Research Overview
- Modeling 3D Humans and Animals
- Human Pose, Shape, and Motion
- Behavior, Action, and Language
- Synthesizing People
- Society, Medicine, and Psychology
- Scenes, Structure and Motion
- Beyond Mocap
- Datasets
- Robot Perception Group
- Holistic Vision Group
- Data Team
-
Completed Projects
- Human Pose, Shape and Action
- 3D Pose from Images
- 2D Pose from Images
- Beyond Motion Capture
- Action and Behavior
- Body Perception
- Body Applications
- Pose and Motion Priors
- Clothing Models (2011-2015)
- Reflectance Filtering
- Learning on Manifolds
- Markerless Animal Motion Capture
- Multi-Camera Capture
- 2D Pose from Optical Flow
- Body Perception
- Neural Prosthetics and Decoding
- Part-based Body Models
- Intrinsic Depth
- Lie Bodies
- Layers, Time and Segmentation
- Understanding Action Recognition (JHMDB)
- Intrinsic Video
- Intrinsic Images
- Action Recognition with Tracking
- Neural Control of Grasping
- Flowing Puppets
- Faces
- Deformable Structures
- Model-based Anthropometry
- Modeling 3D Human Breathing
- Optical flow in the LGN
- FlowCap
- Smooth Loops from Unconstrained Video
- PCA Flow
- Efficient and Scalable Inference
- Motion Blur in Layers
- Facade Segmentation
- Smooth Metric Learning
- Robust PCA
- 3D Recognition
- Object Detection
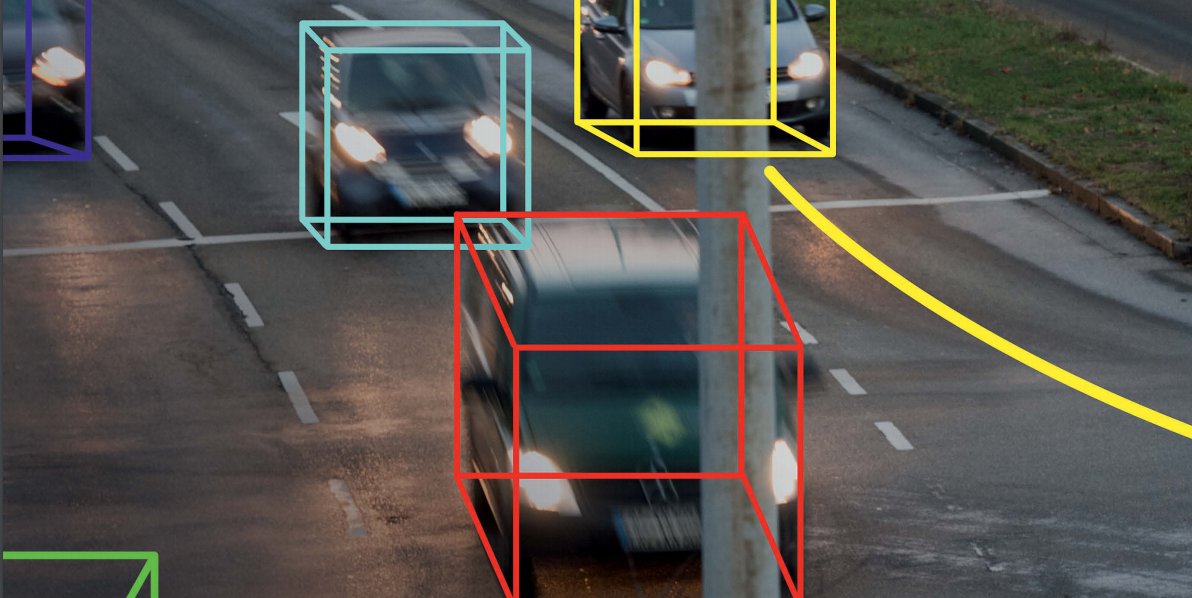
Photo: Wolfram Scheible.
Scene understanding, in contrast to object recognition, attempts to analyze objects in context with respect to the 3D structure of the scene, its layout, and the spatial, functional, and semantic relationships between objects. Our research in this area combines object detection/recognition with 3D reconstruction and spatial reasoning. We believe that the integrated analysis of low-level image features, together with high-level semantic and 3D object models, will enable robust scene understanding in complex and ambiguous environments and will provide the foundation for further reasoning.