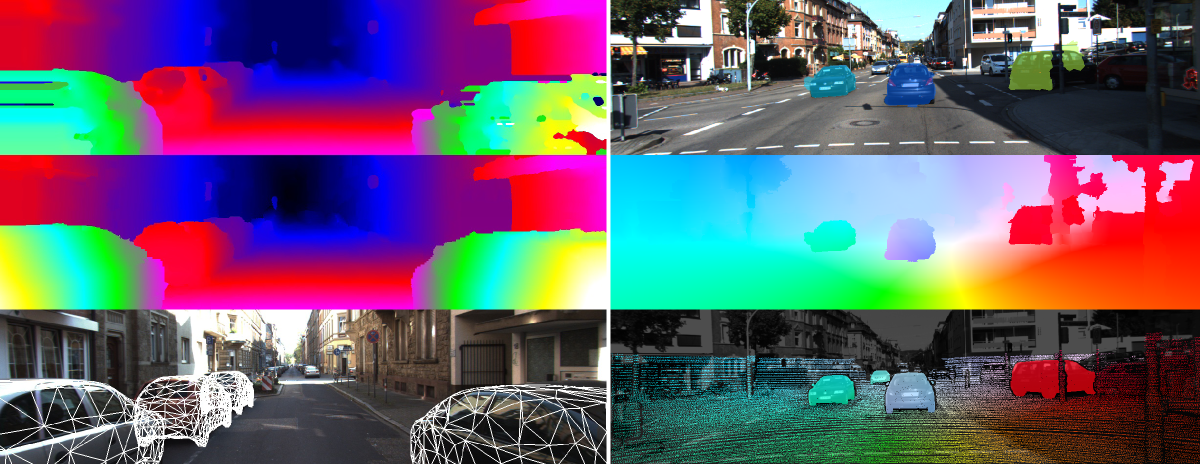
Left: Using object knowledge we are able to resolve stereo ambiguities, in particular at textureless and reflective surfaces. This leads to smoother and more accurate depth maps (middle) compared to using classical local regularizers (top). In additon we obtain highly detailed 3D object estimates (bottom) [ ]. Right: Our 3D scene flow model decomposes the scene into its rigid components. This way, we simultaneously obtain a motion segmentation of the image (top) while implicitly regularizing the scene flow solution (middle) [ ].
While many computer vision problems are formulated as purely bottom-up processes, it is well known that top-down cues play an important role in human perception. But how can we integrate this high-level knowledge into current models? In this project, we investigate this question and propose models for stereo [ ], scene flow [ ], and 3D scene understanding [ ] which formulate our prior belief about the scene in terms of high-order random fields [ ]. We also tackle the aspect of tractable approximate inference which is particularly challenging for these kind of models.
Stereo techniques have witnessed tremendous progress over the last decades, yet some aspects of the problem remain challenging today. Striking examples are reflective and textureless surfaces which cannot easily be recovered using traditional local regularizers. In [ ], we therefore propose to regularize over larger distances using object-category specific disparity proposals. Our model encodes the fact that objects of certain categories are not arbitrarily shaped but typically exhibit regular structures. We integrate this knowledge as non-local regularizer for the challenging object category "car" into a superpixel based CRF framework and demonstrate its benefits on the KITTI stereo evaluation.
In [ ], we propose a novel model and dataset for 3D scene flow estimation with an application to autonomous driving. Taking advantage of the fact that outdoor scenes often decompose into a small number of independently moving objects, we represent each element in the scene by its rigid motion parameters and each superpixel by a 3D plane as well as an index to the corresponding object. This minimal representation increases robustness and leads to a discrete-continuous CRF where the data term decomposes into pairwise potentials between superpixels and objects. Moreover, our model intrinsically segments the scene into its constituting dynamic components. We demonstrate the performance of our model on existing benchmarks as well as a novel realistic dataset with scene flow ground truth. We obtain this dataset by annotating 400 dynamic scenes from the KITTI raw data collection using detailed 3D CAD models for all vehicles in motion. Our experiments also reveal novel challenges which cannot be handled by existing methods.