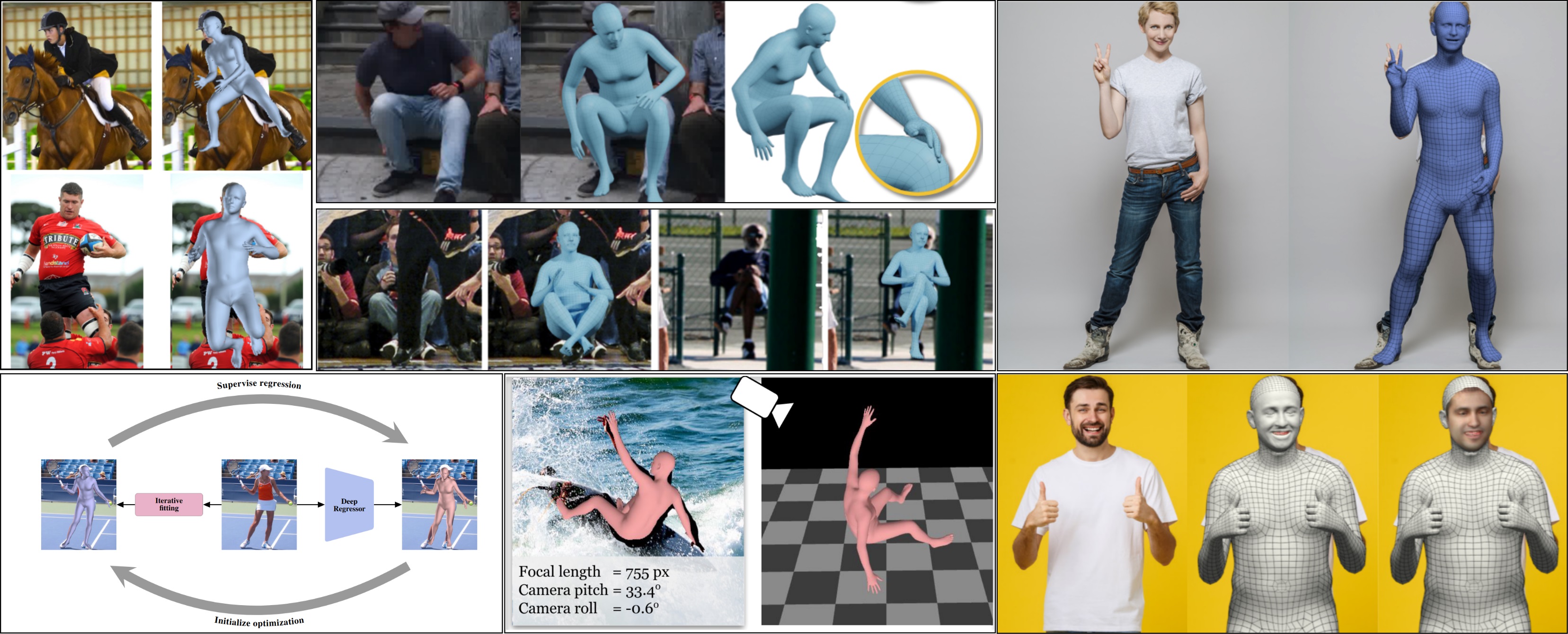
Regression of 3D bodies from images. Left: HMR [ ] (top) and SPIN [ ] (bottom) are foundational methods. Middle: Recent work improves results by considering self-contact (top, TUCH [ ]), training for robustness to occlusions (middle, PARE [ ]), and estimating perspective camera parameters (bottom, SPEC [ ]). Right: We also regress expressive bodies with articulated hands and faces (top, ExPose [ ]), including detailed face shape (bottom, PIXIE [ ]).
Estimating the full 3D human pose and shape (HPS) directly from RGB images enables markerless motion capture and provides the foundation for human behavior analysis. Classical top-down model fitting approaches have several limitations, they require pre-computed keypoints, which are difficult to obtain in complex scenarios and for bodies with occlusions, they are computationally slow (> 30 seconds per image), and these methods are easily trapped in local minima. In contrast, regression methods directly learn the mapping between image pixels and 3D body shape and pose using a deep neural network.
The first HPS regressor, HMR [ ], is trained using only a 2D joint reprojection error by exploiting an adversarial loss that encourages the model to produce SMPL parameters that are indistinguishable from real ones. VIBE [ ] generalizes HMR to videos by using a temporal discriminator learned from AMASS [ ]. SPIN [ ] uses the current regressor to initialize optimization-based fitting, which then serves as supervision to improve the regressor in a collaborative training framework.
Our recent work builds on HMR and SPIN, addressing their limitations. PARE [ ] learns to predict body-part guided attention masks to increase robustness to partial occlusions by leveraging information from neighboring, non-occluded, body-parts. SPEC [ ] learns a network to estimate a perspective camera from the input image, and uses this to regress more accurate 3D bodies. TUCH [ ] augments SPIN during training with 3D bodies that are obtained by exploiting discrete contacts during pose optimization, improving reconstruction performance for both self-contact and non-contact poses.
Typical HPS regressors work in two stages: they detect the human and then regress the body in a cropped image. ROMP [ ] replaces this with a single stage by estimating the likelihood that a body is centered at any image pixel along with a map of SMPL parameters at every pixel. ROMP estimates multiple bodies simultaneously and in real time.
Most methods regress SMPL parameters. ExPose [ ] estimates SMPL-X, including hand pose and facial expression, using body-part specific sub-networks to refine the hand and face parameters with body-driven attention. PIXIE [ ] goes further, introducing a moderator that merges the features of different parts. PIXIE also increases realism by estimating gendered body shapes and detailed face shape.